- AWS
- Data and AI
- Generative AI
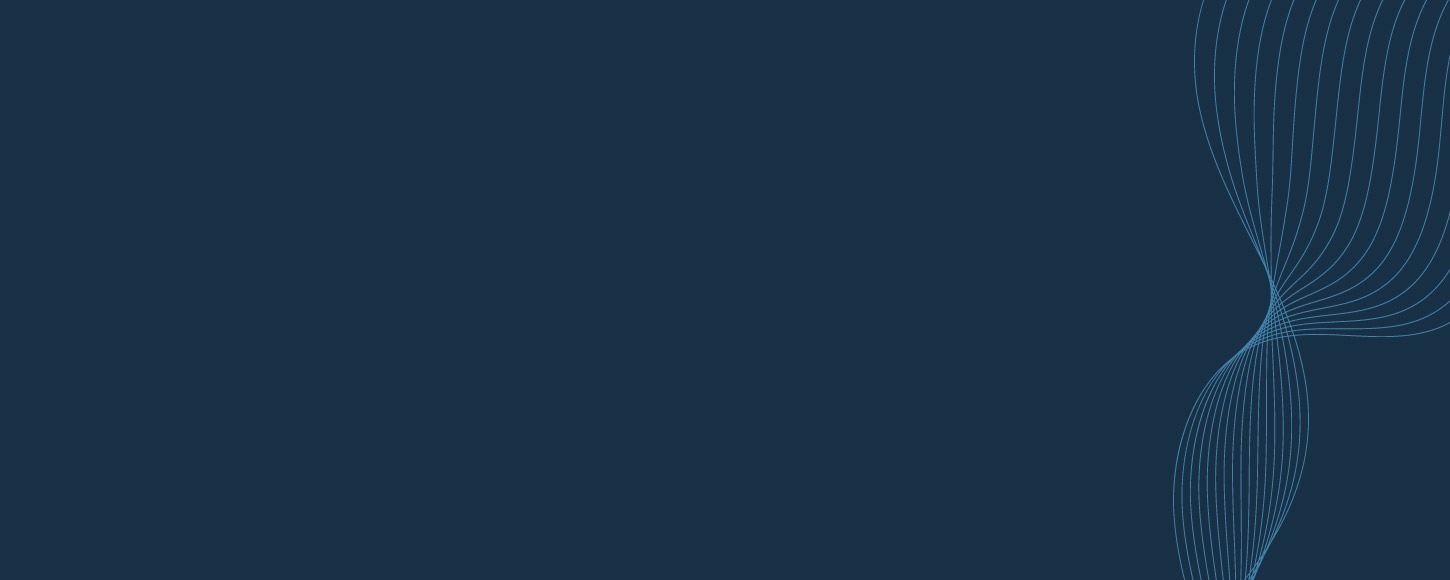
Conquer 2025 with AI: A Practical Guide for Business Leaders
Generative AI has exploded onto the scene, promising to revolutionize business. But hype aside, 2025 is the year for practical AI adoption. Are you ready to move beyond the “wow” factor and unlock real value? This guide provides a strategic roadmap for effectively leveraging AI.
Identify High-Impact AI Opportunities:
Don’t chase trends. Focus on solving your most pressing business challenges. AI excels at:
- Automation: Streamlining repetitive tasks for increased efficiency.
- Data Analysis: Extracting actionable insights from massive datasets.
- Pattern Recognition: Uncovering hidden relationships to drive smarter decisions.
Ask yourself:
- What data do we use most?
- What insights are we currently extracting?
- Where are our process bottlenecks?
Focusing on these questions will help you identify the highest-impact AI opportunities. For example, we helped Alida, a global experience management leader, reduce response analysis time from days to hours using AI-powered natural language processing. This “quantum leap” in sentiment analysis gave Alida real-time insights for improved customer satisfaction. Similarly, we helped MLSE boost fan engagement by creating an AI-driven platform for personalized storytelling around league stats.
Build a Robust Data Foundation for AI Success:
While Large Language Models (LLMs) offer a low barrier to entry, sustainable AI success hinges on your data. Proprietary data is the foundation of truth and context for your AI initiatives. It’s what makes your AI models relevant, accurate, and aligned with your business needs. This is especially true as you move beyond general-purpose LLM chatbots and begin fine-tuning models and customizing them for specific business functions.
Effective data management is crucial. Start by:
- Inventorying Data Sources: Identify all databases, warehouses, and storage locations.
- Prioritizing Data: Focus on the most frequently used, largest, and most recent datasets.
Secure the Future of AI: Responsible Development & Deployment:
AI’s potential is immense, but responsible implementation is paramount. Focus on these key areas:
- Data Security: Integrate security at every stage of the AI lifecycle. Address:
- Data sensitivity (PII, PHI, etc.)
- Access controls
- Security measures (encryption, intrusion detection)
- Regulatory compliance (GDPR, CCPA, HIPAA)
- Model Operations & Output Monitoring: Prioritize model quality over cost-cutting. Implement:
- Rigorous testing (unit, integration, end-to-end)
- Relevant evaluation metrics (F1-score, ROUGE, BLEU)
- MLOps and LLMOps: DevOps tailored to machine learning and AI, providing audit trails, access control, and data lineage for anomaly detection and drift prevention and managing the end-to-end workflows.
- Continuous monitoring: Track model performance in production to identify and address issues like bias, drift, or unexpected outputs.
Success with AI requires a strategic approach: identify high-impact use cases, build a robust data foundation, and prioritize data security and model quality. Continuous learning and a commitment to responsible AI are essential for navigating this dynamic landscape and achieving sustainable success. Contact OpsGuru today to learn how we can help you unlock the power of AI in 2025.
Ready to make measurable business impacts using AI? Contact us to get started.