- Data and AI
- Generative AI
- Innovation
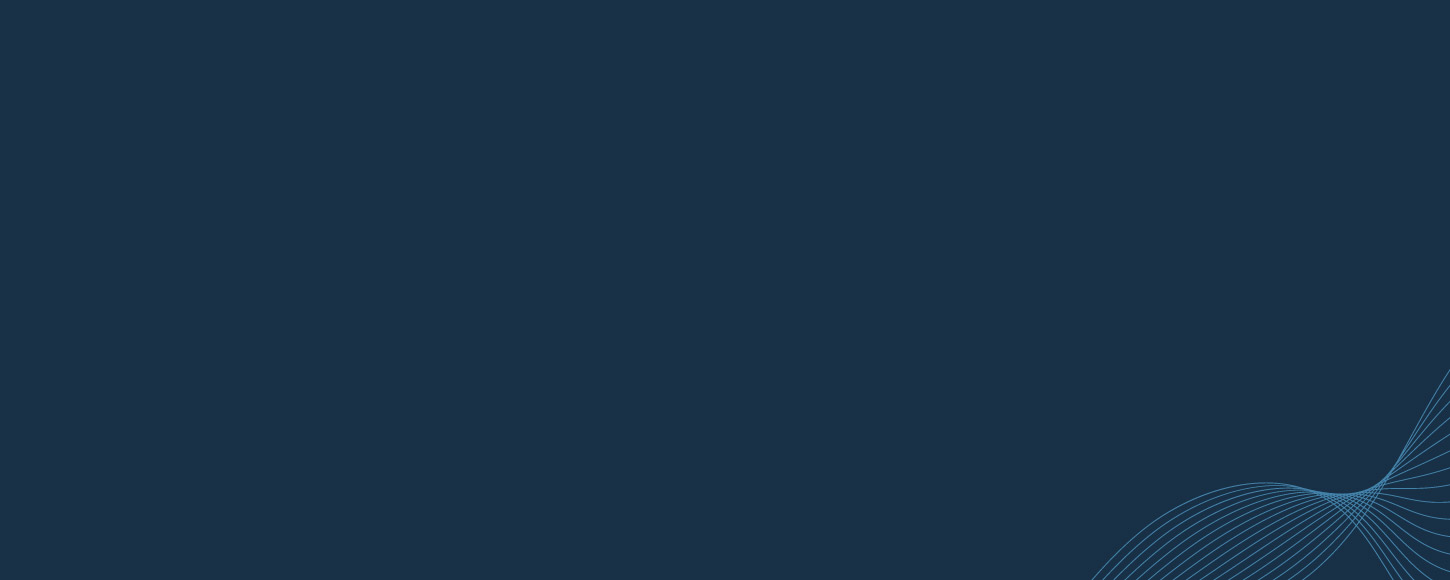
Demystifying AI Agents: 6 Types and Characteristics
The term “AI agent” is generating significant buzz, but with that buzz comes confusion. Some envision AI agents as autonomous entities capable of navigating complex scenarios and making independent decisions. In contrast, others describe them as simple rule-based bots—not much different from traditional automation.
Artificial Intelligence (AI) agents are gaining traction because they can revolutionize how we interact with technology. They are a powerful tool that offers enhanced efficiency, improved decision-making, personalized experiences, scalability, and 24/7 availability. We free up human time and resources for more strategic work by delegating tasks to these intelligent agents.
In this blog post, we’ll explore what AI agents are and the various types you can build to support your business across different industries.
What are AI Agents?
Artificial Intelligence (AI) agents come in various forms, each designed to handle increasing levels of complexity, autonomy, and intelligence. At one end of the spectrum are simple reflex agents: rule-based systems built for automation and predictable responses. As we progress along the spectrum, agents evolve to incorporate context, goals, utility optimization, learning, and coordination.
This progression marks the journey from basic automation to increasingly autonomous and intelligent agent systems. Understanding these different types helps illuminate how AI can be applied in both straightforward tasks and complex problems in dynamic environments. Below, we’ll explore how they pave the way for agentic AI to take centre stage in future applications.
Types of AI Agents
To understand the diverse landscape of AI agents, we’ll explore a framework that categorizes them based on their purpose and capabilities. There are various ways to categorize AI agents that exist:
- Simple reflex agents focusing on Automation
- Model-based reflex agents
- Goal-based agents
- Utility-based agents
- Learning agents
- Hierarchical agents
We use this framework as it provides a clear understanding of the different agent types and their technological implementations.
Simple reflex agents focusing on Automation
Simple reflex agents are the most basic type of AI agent. They operate on predefined rules, making them straightforward to implement. They don’t need generative AI or complex reasoning.
Instead, they follow an “if-then” logic: if a specific event occurs, they perform a predefined action. Technologies like rule engines (e.g., Drools), state machines (e.g., AWS Step Functions), and serverless functions (e.g., AWS Lambda) can be used to build these agents.
A common example is an automated system that sends a welcome email when a new customer signs up.
Model-based reflex agents
Taking a step beyond simple reflexes, these agents incorporate a “world model” to enhance their decision-making. This model, often powered by Large Language Models (LLMs), allows the agent to analyze the context of a situation and make more informed decisions. In the shift to incorporating a “world model” and contextual analysis, we begin to see the nascent form of Agentic AI taking shape.
For example, a customer service chatbot could use an LLM to understand the nuances of a customer’s query and provide a more relevant and helpful response. Personalized recommendation systems and fraud detection systems are other examples of model-based reflex agents.
Goal-based agents
These agents are designed to achieve specific objectives. They use reasoning and planning to determine the best action to reach their goals. A popular technique for implementing goal-based agents is ReAct (Reason and Act), which combines reasoning and action for multi-step problem solving. LLMs can play a crucial role in goal-based agents by helping to represent goals in a structured format, enabling more effective planning and reasoning.
A classic example is a chess-playing AI that analyzes the board and plans its moves to achieve the ultimate goal of checkmating the opponent. Other applications include task automation with complex workflows, robotics, and autonomous navigation.
Utility-based agents
Going beyond simply achieving goals, utility-based agents aim to maximize a specific measure of “goodness” or utility. This utility function can be defined to incorporate various factors and preferences. Retrieval Augmented Generation (RAG) plays a key role here, allowing agents to access and process real-time information to optimize decision-making. ReAct can be adapted to implement utility-based agents by incorporating the utility function and RAG into its reasoning process.
For example, a travel booking agent could use a utility function that considers factors like price, travel time, and comfort to find the best flight for a user. With RAG, the agent can access real-time data on flight availability, prices, and even weather conditions to refine its recommendations.
Learning agents
Learning agents represent a significant step towards autonomous AI, embodying the capacity to evolve and adapt through experience. They can analyze their past actions, identify areas for improvement, and adjust their strategies accordingly. This learning process often involves several key components:
- Memory: Arguably the most essential component, memory allows the agent to store and recall past experiences, including successes, failures, and the outcomes of different actions. This stored knowledge informs future decisions and helps avoid repeating mistakes. Without some form of memory, an agent cannot learn from history.
- Feedback Mechanisms: Crucial for most learning agents, feedback mechanisms provide information about the consequences of actions, enabling the agent to evaluate its performance and identify areas where it can improve. Feedback can come from various sources, including user input, environmental signals, or self-assessment. While most learning agents rely on feedback, certain unsupervised learning methods can learn patterns and structures without explicit feedback.
- Knowledge Base: A knowledge base is a repository of information agents can use to learn and reason about the world. This can include facts, rules, and relationships that help the agent understand its environment and make informed decisions. While not strictly necessary for all learning agents—as some can learn directly from raw data or interactions—a knowledge base can provide valuable prior information and accelerate learning, especially in complex domains.
- Retrieval Augmented Generation (RAG): RAG enables the agent to access and integrate real-time information from external sources into its learning process. This allows the agent to adapt to changing circumstances and make more informed decisions. While not essential for basic learning, RAG enhances the agent’s ability to adapt and evolve in dynamic environments.
Learning agents embody a more mature Agentic AI, surpassing model-based, goal-based, and utility-based agents by learning and adapting. They autonomously refine strategies through memory, feedback, and knowledge, enabling independent action and goal achievement. This self-improvement is key to true autonomy, allowing operation in dynamic environments without constant intervention.
While the level of autonomy varies depending on factors like learning mechanisms, knowledge, and human oversight, learning agents conceptually embody key characteristics associated with autonomous agents, representing a significant step towards realizing truly independent AI systems.
Hierarchical agents
Building upon the concept of individual learning agents, hierarchical agents introduce a new level of sophistication by organizing these agents into coordinated teams. Multi-agent systems break down complex tasks into smaller, more manageable subtasks, delegating them to lower-level agents and orchestrating their efforts to achieve the overall objective. This hierarchical structure mirrors how a well-organized team functions in the real world, with specialized individuals contributing their expertise to a shared goal.
For example, a project management AI could act as a hierarchical agent, delegating tasks to specialized agents for scheduling, communication, and resource allocation. This division of labour allows for greater efficiency and specialization, enabling the system to solve complex challenges.
How Can AI Agents Be Used in Different Types of Industries?
AI agents are versatile. They can be tailored to meet the needs of a wide range of industries, from enhancing operational efficiency to improving customer experiences. These intelligent systems are transforming how businesses operate:
Model-Based Agents in Healthcare
Model-based agents can simulate human reasoning and decision-making, making them ideal for complex environments like healthcare. These AI agents can help diagnose illnesses, personalize treatment plans, and monitor patient health in real-time. By analyzing large sets of medical data, model-based agents assist healthcare professionals in delivering faster, more accurate care.
Utility-Based Agents in Energy & Utilities
In the energy sector, utility-based agents are used in smart grid systems to optimize electricity distribution. These agents make decisions based on maximizing a specific utility, such as energy efficiency or cost savings, while adapting to fluctuating demand and supply conditions. This leads to stable energy delivery, reduced waste, and lower costs.
We go into more examples in our blog post on Autonomous Systems here.
AI Agents in Customer Support
Customer service has been one of the most prominent areas for AI agent adoption. From virtual assistants to intelligent chatbots, AI agents can handle routine inquiries, provide 24/7 support, and escalate complex issues to human agents when needed. This not only improves customer satisfaction but also reduces the workload for support teams.
Amazon Bedrock Agents: The Technology Behind Intelligent AI Agents
Understanding the diverse capabilities of AI agents is crucial for making informed decisions about their design and deployment. Different frameworks and approaches exist because of the diverse array of use cases and their specific requirements. By understanding the strengths and limitations of each type of agent, we can select the right one for a particular task and ensure that AI is used responsibly and beneficially.
Amazon Bedrock is a fully managed service that makes building and scaling generative AI applications easy. It offers a wide range of foundation models (FMs) for text and images, allowing you to find the model that best suits your needs. You can even customize these FMs with your own data, and easily integrate them into your applications using familiar AWS tools.
In our next blog, we’ll dive deeper into the technical aspects of AI agents, exploring how Amazon Bedrock and other technologies can be used to implement these different types of agents. Read on to learn how to bring these agents to life and harness their full potential for your business.